Please, Let’s Stop the Epidemic of Armchair Epidemiology
Ignore the people misconstruing their expertise and offering false certainty.
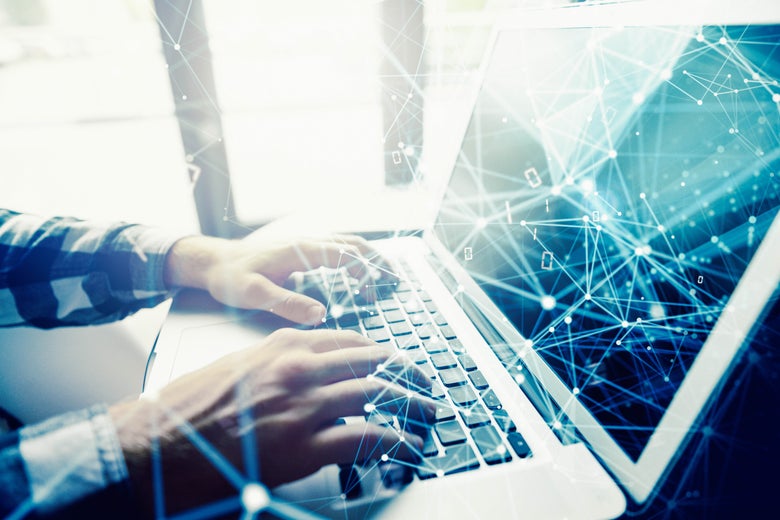
On Friday, a Silicon Valley technologist named Aaron Ginn self-published an article on Medium called “Evidence Over hysteria—COVID-19” that garnered millions of views. Touting his marketing expertise in “understanding virality,” Ginn gathered publicly available epidemiological data to make the case that the coronavirus threat to the U.S. doesn’t merit the dramatic response called for by public health officials: “Shuttering the local economy is a distraction and arbitrary with limited accretive gain outside of greatly annoying millions and bankrupting hundreds of businesses.”
Ginn’s analysis certainly had a seductive contrarian appeal—after all, who wouldn’t want to hear that the pandemic won’t be as bad as we were told? But a closer look by scientists revealed his number-crunching to be riddled with inconsistencies and entry-level errors. Over the weekend, Medium took down the post.
Ginn is far from the only quantitative type posting superficially convincing but flawed epidemiological analyses of the ongoing global pandemic. Across social media, Silicon Valley data wonks, as well as people with Ph.D.s in fields tangentially related to epidemiology, are analyzing public datasets, posting graphs, and making sweeping predictions about the pandemic. Some, like Ginn, draw conclusions that contradict accepted public health advice. Other casual assessments of the numbers are being used to make uselessly terrifying cases for containing the virus’s spread. Wherever you look, people have become suspiciously comfortable with concepts like R0 and CFRs, which they use to argue in favor of or against widespread social distancing. At least one epidemiologist has called the phenomenon a crisis in its own right—an “epidemic of armchair epidemiology.”
One reason back-of-the-envelope hot takes are thriving is that the science of ongoing epidemics is inherently uncertain. And in the U.S., the testing fiasco has only amplified that uncertainty. How many people have the new coronavirus? How many people will become infected and never know? How likely are they to spread it? What role do children play? What is the actual death rate? These are crucial data, and we simply don’t have reliable estimates right now. In addition, how societies behave feeds back into the epidemic’s severity, adding another layer of uncertainty. The way the virus behaves in China or Italy will be different from how it behaves here, depending on what we do. The only thing we know for sure is that things continue to change rapidly, which also means that in these circumstances, anyone claiming certainty is suspect.
I used to be an experimental neuroscientist, so while I’m not a math whiz, I’m comfortable with numbers. I only bring up my background because I understand the temptation to play around with public data. As we all watch an unpredictable crisis unfold in real time, numbers offer refuge in the hell pit of media noise and genuine political turmoil. Perhaps by crunching the numbers myself, I’ll find clarity, or provide helpful information, or find something that challenges accepted wisdom, in a useful way. Plus, playing with numbers is just kind of fun. But should science-minded nonepidemiologist like myself give in to the impulse to post off-the-cuff analyses? Does this add to the discussion, or just provide another source of misinformation with a potentially dangerous veneer of authority?
When Medium took down Ginn’s post (it’s since been posted here), the Wall Street Journal’s editorial board framed its removal as an attempt to “stamp out” free debate and “require conformity with the judgment of expert institutions.” The board has a point: We shouldn’t always blindly trust expertise. Consensus is not always coterminous with truth. And in some cases, outsider viewpoints provide valuable insights that evade insider experts. But this wave of nouveau epidemiologists parsing publicly available data about the new coronavirus does not appear to be one of those cases. Epidemiological amateurs make faulty assumptions, get basic principles wrong, or just pull numbers out of thin air. Slipshod data-crunching doesn’t challenge the consensus in any meaningful way. Opining with numbers just because you use numbers in your day job isn’t formulating a rigorous dissent; it’s overestimating your abilities while lacking self-awareness of your own incompetence—the Dunning-Kruger effect. In my experience, the Dunning-Kruger effect seems particularly strong in people who think real expertise in one area automatically confers expertise in another.
“I’m quite experienced at understanding virality, how things grow, and data,” Ginn writes, in a hall-of-mirrors move that seems to elide the fact that marketing virality is a bastardized metaphor cribbed from … epidemiology. “In my vocation, I’m most known for popularizing the ‘growth hacking movement’ in Silicon Valley that specializes in driving rapid and viral adoption of technology products. Data is data. Our focus here isn’t treatments but numbers. You don’t need a special degree to understand what the data says and doesn’t say. Numbers are universal.” That has a nice ring to it. It’s also not true.
Data isn’t just data. It can’t exist without context. Rather than diving into Ginn’s analysis, which is lengthy and complicated and has already been refuted in some detail by more qualified critics, let me offer a simple example of how a lack of expertise can make analyses go awry. Last week, I came across a Medium post by another data scientist named Abe Stanway. Stanway wanted to figure out how to track the outbreak in New York City when testing was almost nonexistent—a laudable goal. So he ran some numbers using New York City’s publicly available data on influenzalike illness, which tallies emergency room visits where the chief complaint mentions flu, fever, and sore throat. Stanway reasoned unusual upticks in visits by patients complaining of these symptoms might reflect unknown COVID-19 cases. One of his most surprising conclusions was that the epicenter of the New York City outbreak was a Queens neighborhood called, believe it or not, Corona. Stanway tweeted his discovery (“You just can’t make this shit up”). I noticed this tweet and his analysis concerned me because my child care provider, who is nearly 60 years old, lives near that neighborhood. I thought it might be worth giving her a heads up to be extra careful.
I also thought I’d check over Stanway’s work before potentially panicking our child care provider. And, I’ll admit, I was eager to peruse the data. So I went to the NYC Health Data site, got to work, and immediately found a likely flaw in Stanway’s method. Stanway was correct that, at the time of his analysis at least, the Corona neighborhood had the highest number of reported cases in NYC. But by comparing this year’s data with previous years’ data, I noticed the Corona neighborhood has the highest number of flu cases every year. That suggests this year’s high rate reflects baseline characteristics of the neighborhood—such as a population more likely to go to the emergency room for health care—rather than indicating that it’s the epicenter of this particular outbreak. Sure, it may be possible that Corona has special characteristics and it’s the center of the current coronavirus outbreak. I don’t know. But the point is that neither does Abe Stanway. It takes knowledge to know what other variables might be thwarting your analysis. But Stanway didn’t take any steps to control for confounding variables. I asked an epidemiologist at NYU to check over my reasoning, and he confirmed that one of the first things you’d want to do is compare this year with previous years, to understand the baseline for the area. In other words, Stanway’s omission is a mistake few experts—who would understand the problem of likely confounders—would make.
This isn’t to pick on Stanway (although, like many others, he should take down his misleading post), but to point out a larger problem with coronavirus data vigilantes: They don’t know what they don’t know. They are oddly comfortable marshalling irrelevant credentials to make sweeping predictions that muddy the waters at a profoundly critical time. As one Twitter user quipped, “Coronavirus can cause a hacking cough. As a software engineer, I know a thing or two about hacking. In this Medium post I will be.”
Stanway, it seems, was just having fun with the numbers, and perhaps thought his conclusions would be helpful to someone. But Ginn—whose Medium post gained far more attention—had a clearer purpose: to persuade. This is a crucial distinction. Ginn is making two types of arguments: a scientific one (what is happening with the pandemic?) and a political one (what should we do about it?). These questions are of course interrelated. But the political question, in particular, urgently needs to be addressed, even though it’s often something public health officials like to evade.
By presenting his argument as fundamentally scientific, Ginn comes off as an objective, just-the-facts guy, someone with expertise that readers searching for answers can trust. But this coy stance is a rhetorical one that helps advance a political argument. At the end of his post, when Ginn debates the trade-offs of various political responses, I don’t actually disagree with many of the points he raises. The economic consequences of mitigating the pandemic are huge. The powers gained by the state in this situation are troubling. The government authorities making these decisions should absolutely be questioned and subjected to intense scrutiny. Ginn is right to push back, but the problem is how he does it. His bizarre credentialing flex and subsequent numerical legerdemain—which at first glance seem to boost his credibility—ultimately make his pushback seem less reliable.
These posts may be coming in response to an epidemic, but they reflect the alternative facts problem endemic to American political psychology—just now with a pernicious quantitative twist. As public opinion about the appropriate response to the pandemic becomes—predictably and depressingly—hyperpolarized, people are falling in line. There’s a growing movement in conservative circles to end the shelter-in-place directives and a growing insistence in liberal circles to keep them in place. Earlier this week, trending on Twitter were #ReopenAmerica (among conservatives) and #NotDyingForWallStreet (among liberals). With the help of the president, we’re watching the conversation devolve into a false dichotomy between saving the economy and preserving public health. In this escalating din, I hear the familiar refrains of the culture wars. Posts like Ginn’s entrench each side further, because now each side gets to claim its own set of settled “facts.” In reality, a rapidly developing pandemic requires we tolerate some amount of uncertainty.
That said, the facts on the ground make it clear that many things are far less uncertain than the raft of Medium posts might lead the casual reader to believe. Countries that have failed to take preventive measures get hit harder. Health care systems in New York City are already becoming overwhelmed before the pandemic has peaked. It’s necessary and important to debate the political response to the pandemic. But as a few researchers penned in their own cheeky Medium post, we need to flatten the curve of armchair epidemiology (“If take appears hot/feverish, seek expert help”). When the stakes are this high, the decision to use your authority (and yes, having a Ph.D. or a fancy job title is a form of authority) is an ethical one: Just because you can analyze data doesn’t mean you should.
For more on the impact of the coronavirus, listen to the latest episode of What Next.
Support our independent journalism
Readers like you make our work possible. Help us continue to provide the reporting, commentary, and criticism you won’t find anywhere else.
Follow Us!